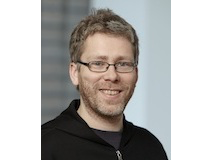
Marc Pollifeys
ETH, Zurich | Title: TBA
Abstract: TBA
|

Kai Yu
Horizon Robotics Inc., China | Title: High-performance object detection with a dense prediction map and its application to ADAS
Abstract: Object detection is an important computer vision problem for a large variety of applications. In camera-based sensing systems for intelligent vehicles, object detection offers the fundamental ability to real-time environment perception. Recently, convolutional neural networks (CNNs) have led to significant improvements in this area. However, the current state-of-the-art CNN-based detection methods (R-CNNs) still suffer from low efficiency and pool accuracy on car detection tasks, in which objects usually are small and heavily occluded. In this talk, I will present an end-to-end object detection method, which archives the best performance on KITTI car detection task. Our small network can run at real-time on KITTI images, and still outperformance Faster R-CNN using VGG16.
Bio:
Dr. Kai YU is the founder & CEO of Horizon Robotics Inc., a Beijing-based startup dedicated to developing artificial intelligent chips and software platforms for autonomous driving and smart home. He led the R&D of AI at Baidu from April 2012 to June 2015. He founded Baidu IDL (Institute of Deep Learning), the very first and most renowned AI lab in China industry. His team developed cutting-edge technologies to transform voice search, computer vision, online advertising and web search, and unprecedentedly won the Baidu Highest Achievement Award for 3 times. In 2013, Dr. Yu also launched the first autonomous driving project in China, which later became the Baidu autonomous driving BU (business unit). Dr. Yu has published around 60 papers with more than 11000 citations. In 2011 as an adjunct faculty, he taught a class “CS121: Introduction to Artificial Intelligence" at the Computer Sciences Dept. of Stanford University. He has received many awards, including the Best Paper Runner-up Award of ICML-2013, the First Place of PASCAL VOC 2009,and the First Place of ImageNet Challenge 2010. Before joining Baidu, he was a Department Head of NEC Labs America, and a Senior Research Scientist at Siemens. He received his B.Sc and M.Sc degrees in E&E from Nanjing University, China, and a doctorate degree in Computer Science from University of Munich, Germany.
|

Jianxiong Xiao
Princeton, EEUU
| Title: Deep Learning for Autonomous Driving: What is beyond detection, segmentation, and control?
Abstract: Deep learning has revolutionized computer vision and has a huge potential impact for autonomous driving. Typical ways of deploying deep learning tools in autonomous driving is to use convolutional neural networks for object detection and semantic segmentation in streetview images. Recently, Muller et al. from NVIDIA also demonstrated impressive results to use deep ConvNets to regress steering wheel control. In this talk, beyond these, we argue that it is much broader and deeper what deep learning can do for autonomous driving.
First, I will present Deep Driving, a system that learns affordance indicators for direct perception, such as the distance to the car in front. Training with the data recorded from human-playing of a video game for a few hours, we build a Level-4 system to master car racing in the game. Second, I will present Invisible Map, a system that exploits the huge amount of streetview images available online to unsupervisedly learn to extract critical information from an image that a map would tell for autonomous driving, in order to get rid of dependency for maps. Third, I will present some parts of the research we have been working on at AutoX.
Bio:
Jianxiong Xiao (a.k.a., Professor X) is the Founder and CEO of a high-tech startup AutoX (currently in stealth mode). Previously, he was an Assistant Professor in the Department of Computer Science at Princeton University and the founding director of the Princeton Computer Vision and Robotics Labs from 2013 to 2016. He received his Ph.D. from the Computer Science and Artificial Intelligence Laboratory (CSAIL) at the Massachusetts Institute of Technology (MIT) in 2013. Before that, he received a BEng. and MPhil. in Computer Science from the Hong Kong University of Science and Technology in 2009. His research focuses on bridging the gap between computer vision and robotics by building extremely robust and dependable computer vision systems for robot perception. In particular, he is a pioneer in the fields of 3D Deep Learning, Autonomous Driving, RGB-D Recognition and Mapping, Big Data, Large-scale Crowdsourcing, and Deep Learning for Robotics. His work has received the Best Student Paper Award at the European Conference on Computer Vision (ECCV) in 2012 and the Google Research Best Papers Award for 2012, and has appeared in the popular press. Jianxiong was awarded the Google U.S./Canada Fellowship in Computer Vision in 2012, the MIT CSW Best Research Award in 2011, and two Google Faculty Awards in 2014 and in 2015 respectively. More information can be found at: http://www.jianxiongxiao.com
|

Urs Muller
NVIDIA self-driving cars, USA | Title: End-to-end Learning for Autonomous Driving
Abstract: The presentation starts with a general overview of NVIDIA tools for autonomous driving. Subsequently, an architecture and training methods are presented which were used to build an autonomous road following system. A key aspect of the presented approach is eliminating the need for hand-programmed rules and procedures - such as finding lane markings, guardrails or other cars - thereby avoiding the creation of a large number of "if, then, else" statements. The system learns to map the pixels of a single front-facing camera directly to steering commands without explicit lane detection and path planning. It can drive on various road types from highway to unpaved roads under various lighting and weather conditions.
|

Daniel Cremers
Technische Universität München | Title: Dense & Direct Visual SLAM for Autonomous Quadcopters
Abstract: The reconstruction of the 3D world from images is among the central challenges in computer vision. Starting in the 2000s, researchers have pioneered algorithms which can reconstruct camera motion and sparse feature-points in real-time. In my talk, I will show that one can autonomously fly quadrotors and reconstruct their environment using onboard color or RGB-D cameras. In particular, I will introduce spatially dense methods for camera tracking and reconstruction which do not require feature point estimation, which exploit all available input data and which recover dense or semi-dense geometry rather than sparse point clouds.
This is joint work with Jakob Engel, Jan Stuehmer, Vlad Usenko, Lukas von Stumberg, Christian Kerl and Juergen Sturm.
|

James Peng
Autonomous Driving, Baidu | Title: Baidu’s Endeavor towards Level 4 Autonomous Driving
Abstract: This talk presents Baidu’s strategy and technical endeavor in bringing level 4 autonomous driving into reality. We have ambitious strategic goal of commercializing self-driving in 3 years and mass production in 5 years. We believe that Baidu has many advantages in developing autonomous driving due to our technical advancements in many areas such as deep learning, big data, and high-definition maps. We will present many technical challenges in making our autonomous vehicles coping with China’s unique and diversified traffic conditions. Our big-data and artificial intelligence driven solution to autonomous driving will also be discussed.
James Peng – Chief Architect
Dr. James Peng is Chief Architect at Baidu, and he is in charge of the overall technical directions for autonomous driving. He previously steered the engineering direction for several divisions within Baidu, including monetization platforms, infrastructure department, and data science and big data platform. The projects that he initiated and led have made significant contributions to a wide range of core products. Before joining Baidu, James was at Google Mountain View engineering team. James holds a B.S. degree from Tsinghua University, a M.S. degree from Stats University of New York at Buffalo, and a Ph.D. degree from Stanford University.Kind regards,
Yuan yuan
|